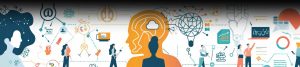
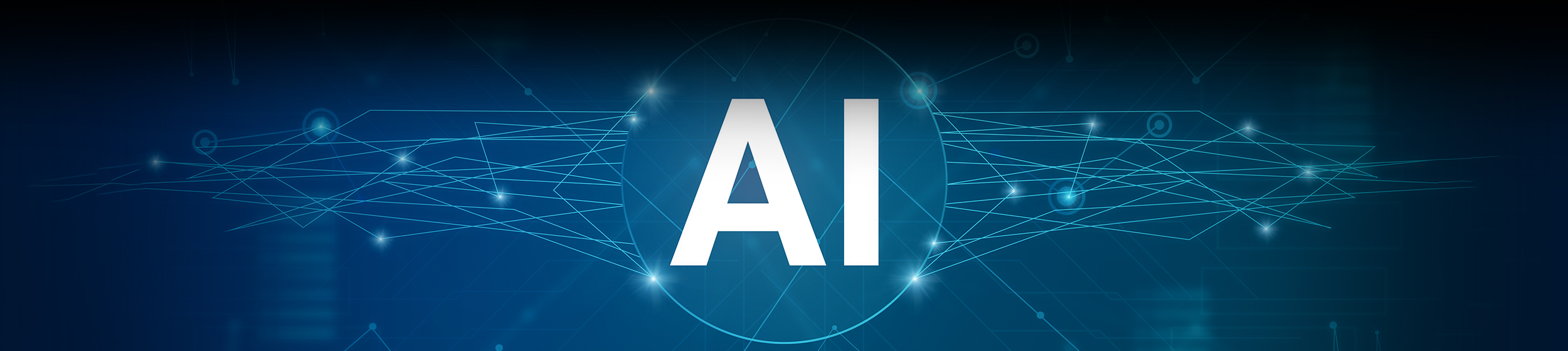
N. Kranthi Kumar
The Role of AI & ML in Guiding Generative AI's Future
The world is getting ready for Generative AI, and Large Language Models (LLMs) are playing a leading role in this shift. Thanks to the familiarity brought by ChatGPT, the public is increasingly adapting to AI and Generative AI in everyday applications. However, while LLMs have immense potential, they also have limitations.
Imagine robots equipped with LLMs that can access and understand maintenance manuals, translating them into actionable steps for engineers. Can we see LLMs analyse the live data from the machines to understand what is going wrong and be able to suggest the engineer to diagnose the machine?
While LLMs are powerful in understanding language and text, they lack the ability to analyse enormous amounts of data needed to predict future actions.
What are Large Language Models (LLMs)?
LLMs, short for Large Language models, are a type of artificial intelligence (AI) trained on enormous amounts of data from public databases that excel at processing and generating human-like language. LLMs can perform various tasks, from translating languages to writing different creative text formats like poems, code, scripts, musical pieces, emails, letters, etc.
The Rise of Large Language Models
The journey of LLMs began with statistical language models, which analyzed text to predict the next word in a sequence based on the statistical analysis of the data provided. These early models were limited in their capabilities and vocabulary. However, advancements in deep learning, particularly the Transformer architecture in 2017, paved the way for the emergence of powerful LLMs.
The LLM landscape is constantly evolving, with a push for larger and more sophisticated models. This is driven by the belief that larger models, trained on more extensive datasets, lead to better performance. The well-known ChatGPT runs on the GPT-3.5 Turbo language model, trained on 175 billion parameters and massive datasets. Lesser-known predecessors like GPT2 and BERT paved the way for modern LLMs. The market now offers several LLM models, including GPT-3.5, GPT-4, PaLM, Gemini, Llama, Mistral, Granite, Falcon, and many others.
Limitations of LLMs
Bias and Fairness
Training on massive datasets raises concerns about bias, fairness, and the potential for perpetuating harmful stereotypes. Additionally, the sheer volume of data required raises ethical questions about data collection and privacy.
Explainability
The internal workings of LLMs are often opaque, making it difficult to understand why they generate specific outputs.
Context-Length Limitations
Most LLMs have limited context length, restricting their ability to handle longer narratives and complex documents and answer questions requiring broader understanding.
Understanding Non-Text Inputs
LLMs struggle to interpret non-textual data like images, tables, and graphs, hindering their ability to address real-world applications that provide input in various formats.
Mitigate Limitations of LLMs with Artificial Intelligence & Machine Learning
While LLMs hold immense potential, their limitations restrict their wider application. Here’s how AI/ML and analytics can come to the rescue, extending their reach:
AI/ML-enabled LLM system for Revolutionizing Maintenance Processes
We previously introduced a challenge faced in a manufacturing scenario. Let’s explore how AI/ ML can empower LLM powered systems tackle this problem effectively
Sensor data integration
Integrate various sensors into the machine to collect real-time data on factors like temperature, vibration, and pressure.
AI/ML for anomaly detection
Train AI/ML models on historical sensor data to identify patterns and deviations indicative of potential malfunctions.
LLM interpretation of anomalies
When an anomaly is detected, the AI/ML model can alert the LLM. The LLM can then access relevant maintenance manuals and historical repair data, combining it with the sensor data to:
Identify potential causes
Analyse the anomaly data and historical information to suggest probable causes of the malfunction.
Recommend diagnostic steps
Based on the identified causes and the maintenance manuals, the LLM can recommend specific diagnostic tests the engineer can perform to pinpoint the exact issue.
Conclusion
In conclusion, transitioning from 4G to 5G represents a paradigm shift in network infrastructure characterized by the adoption of advanced technologies, architectures, and capabilities. From the radio access network to the core network and spectrum utilization to network management, every aspect of network infrastructure transforms to support the demands of the 5G era. As we continue to witness the rollout of 5G networks worldwide, these advancements promise to revolutionize connectivity and unlock new possibilities across industries and sectors.
Related Insights
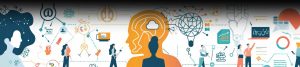