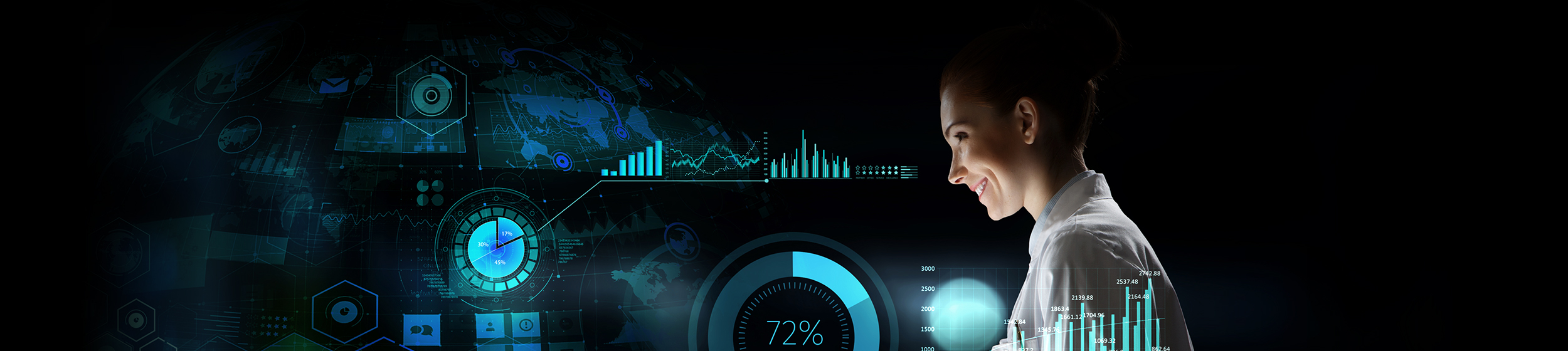
ACL Digital
5 Minutes read
The Impact of Artificial Intelligence on Clinical Data Research Management
Today, we will explore the transformative role of Artificial Intelligence (AI) in clinical data management (CDM) within the life sciences industry, highlighting how AI enhances CDM efficiency, accuracy, and scalability as a critical business function. AI delivers a range of benefits that enhance and optimize data management measures. These benefits include curtailing the time needed to submit queries, speeding up the timeline for database lock, and automating and streamlining standard data management tasks. It authorizes data managers to devote more attention to complex, high-value queries. Further, AI solutions are fully scalable, enabling seamless integration across an entire portfolio.
The use of AI in clinical trials, through its predictive analytics, natural language processing, and capability to monitor data in real-time, can bring down expenses, significantly improve efficiency, and boost data quality. Nevertheless, obstacles like the need for standardization and the integration of AI with current systems prevail.
Integrating AI into clinical data research management has modified clinical trials, improving efficiency, accuracy, and decision-making abilities. Here, we explore the transformative impact of AI and ML in clinical data management, emphasizing how enterprises can leverage this progress through comprehensive Clinical Data Management services and solutions. In summary, AI offers significant potential to improve the effectiveness of clinical trials and focus on patient needs.
Current Market Size and Growth
AI in the healthcare and life sciences market is growing at an impressive pace and is expected to reach about $194.4 billion by 2030. A significant portion of this market is dedicated to improving clinical trials. The area specifically focused on using AI for clinical trials is anticipated to see a compound annual growth rate (CAGR) of around 21% over the same period.
What’s driving this growth? This is mainly due to the increasing use of predictive analytics, machine learning, and natural language processing technologies. These tools are invaluable in managing clinical data more effectively, making processes smoother and more efficient. As a result, life sciences companies that invest in AI recognize the tangible benefits it brings to their operations, positioning themselves for success in the future.
Enhanced Data Collection and Processing
Artificial intelligence-based clinical data management systems have drastically enhanced data collection and processing. Traditionally, managing clinical trial data was labor-intensive and prone to human error. Yet, AI-powered systems now facilitate the seamless extraction and integration of vast amounts of data from various sources, including electronic health records (EHRs), lab results, and patient-reported outcomes.
AI algorithms can determine and correct data discrepancies, assuring high accuracy levels. Machine Learning models also improve this process by learning from data patterns and predicting potential data issues before they arise. This farsighted approach has reduced data processing time and improved the dependability of Clinical Research Management services.
Improved Data Accuracy and Integrity
Data accuracy is paramount in clinical research. Any inaccuracies can lead to flawed results, compromising the entire study and, ultimately, patient safety. AI in clinical data management ensures that data integrity is maintained throughout the research process. By utilizing Natural Language Processing (NLP) and advanced analytics, AI can extract meaningful insights from unstructured data, transforming it into structured formats suitable for analysis.
Clinical Data Management solutions leveraging AI can detect outliers and anomalies in real time, flagging potential data errors for immediate review. This real-time monitoring reduces the need for extensive manual oversight and ensures that data quality remains consistently high. Additionally, AI-powered data validation tools are now essential for Clinical Trial Services, ensuring that studies meet stringent regulatory requirements.
AI algorithms help maintain compliance with data integrity regulations, including Good Clinical Practice (GCP) and FDA’s 21 CFR Part 11 guidelines. AI-powered validation and anomaly detection contribute to a reduction of up to 60% in manual error checks.
Accelerated Clinical Trial Timelines
The capability of AI and ML to facilitate data management processes has led to more instantaneous clinical trial timelines. Clinical Trial Services incorporating AI-based solutions can now process and analyze data at exceptional speeds. For example, AI-driven automation can quickly determine patient eligibility for trials by screening through EHRs and medical histories, which would otherwise take weeks or months.
Similarly, AI tools can optimize patient recruitment and retention, two critical factors that have historically posed significant challenges in clinical trials. By predicting patient drop-out rates and suggesting personalized engagement strategies, AI ensures that trials proceed smoothly, minimizing costly delays. This efficiency level is instrumental for companies seeking reliable Clinical Research Management solutions that can meet market demands.
Enhanced Decision-Making with Predictive Analytics
Predictive analytics, powered by AI and ML, is changing decision-making in clinical data management. AI models can examine historical and real-time data to predict trial outcomes, enabling researchers to make knowledgeable protocol adjustments. This ability lowers risks associated with trial failures and improves the likelihood of accomplishing desired results. Additionally, predictive analytics authorizes researchers to simulate various trial scenarios, optimizing resource allocation and strategic planning. This technology is now integral to Clinical Data Management services that prioritize efficiency and effectiveness.
Cost Reduction and Resource Optimization
One of the most effective benefits of implementing AI in clinical data management is reducing costs. Manual data processing and analysis are resource-intensive and time-consuming. By automating these tasks, AI ML significantly cuts labor expenses and operational costs in clinical data management. Companies leveraging AI in clinical trials report a return on investment (ROI) of up to 400% due to cost reductions and operational efficiencies.
Clinical Data Management solutions powered by AI allow organizations to optimize resource use by automating repetitive tasks and reallocating human resources to more strategic roles. Optimizing these processes reduces overheads, enhancing Clinical Research Management services’ overall productivity and scalability.
Common Challenges in Clinical Data Management
Clinical Data management faces significant hurdles, particularly as the reliance on Electronic Data Capture (EDC) systems has diminished. Just a decade ago, it was common for around 95% of clinical trial data to be recorded within EDC systems, allowing established processes to efficiently manage, review, and monitor the data generated from clinical trials.
However, recent studies indicate a notable shift, with only 30% to 60% of clinical trial data now being collected through EDC systems. This change has led to the emergence of four key challenges in the realms of data collection, monitoring, and management:
Data Chaos
The collection and integration of data from diverse, non-EDC sources has become resource-intensive due to the proliferation of varied data types and large time-series datasets.
Limited Self-Service Access
Data Managers often need help accessing and transforming the necessary data from these various sources, hindering their ability to generate timely and actionable insights for decision-making.
Multiple Roles for Single Tasks
In clinical trial management, completing individual tasks typically involves several roles and skill sets, leading to inefficiencies and delays in task execution.
Scaling Limitations of Manual Processes
As data volumes and complexity increase, manual processes can become unmanageable. Managing exception listings containing thousands of entries can take time, as it renders large-scale data queries and reviews highly impractical.
Addressing these challenges is crucial for improving the efficiency and effectiveness of data management in clinical trials.
Prospects of AI in Clinical Data Management
The future of Clinical Data Management solutions is closely tied to advancements in AI and machine learning technologies. By 2030, AI could enable 50% of clinical trials to incorporate precision medicine protocols, allowing researchers to customize treatments and improve trial success rates. Innovations like deep learning and sophisticated data analytics are set to enhance the accuracy and efficiency of clinical research management processes. Besides, the rise of AI-driven Clinical Trial Services aims to accelerate drug development, making it faster, safer, and more effective.
Moreover, as AI technologies evolve, they will play a pivotal role in personalized medicine, tailoring treatment plans and trials to individual patient profiles. This shift will enhance the efficacy of clinical research and pave the way for more patient-centric approaches.
Conclusion
Integrating AI and ML in clinical data management has diverted the paradigm of clinical trials. From improving data accuracy and accelerating trial timelines to reducing costs and optimizing resources, AI-powered solutions offer considerable benefits that can drive business growth and innovation. Organizations embracing these technologies through robust Clinical Data Management services and Clinical Research Management solutions are better positioned to stay competitive in an ever-evolving healthcare landscape.
The future holds immense potential for AI ML in clinical data management, promising continued enhancements in efficiency, accuracy, and overall quality of clinical research. For companies looking to gain an edge, investing in these advanced technologies is not just an option, it’s a necessity.
Further Reading
Further Reading
- https://www.medidata.com/en/life-science-resources/medidata-blog/ai-in-clinical-data-management/
- https://www.sciencedirect.com/science/article/pii/S2352914817301247
- https://www.syneoshealth.com/insights-hub/ai-and-machine-learning%E2%80%99s-role-clinical-data-management
- https://www.lindushealth.com/blog/the-impact-of-artificial-intelligence-ai-on-clinical-research-a-comprehensive-guide
Related Insights
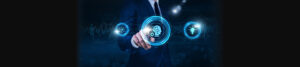
How AI Agents will Drive Us from Automation to Autonomy
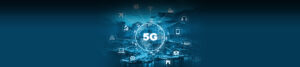
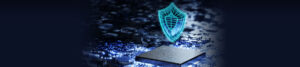
Best Practices to Secure Databases
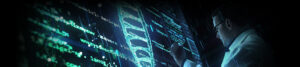
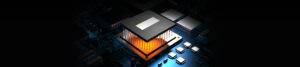
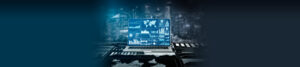