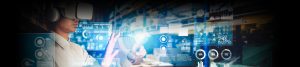
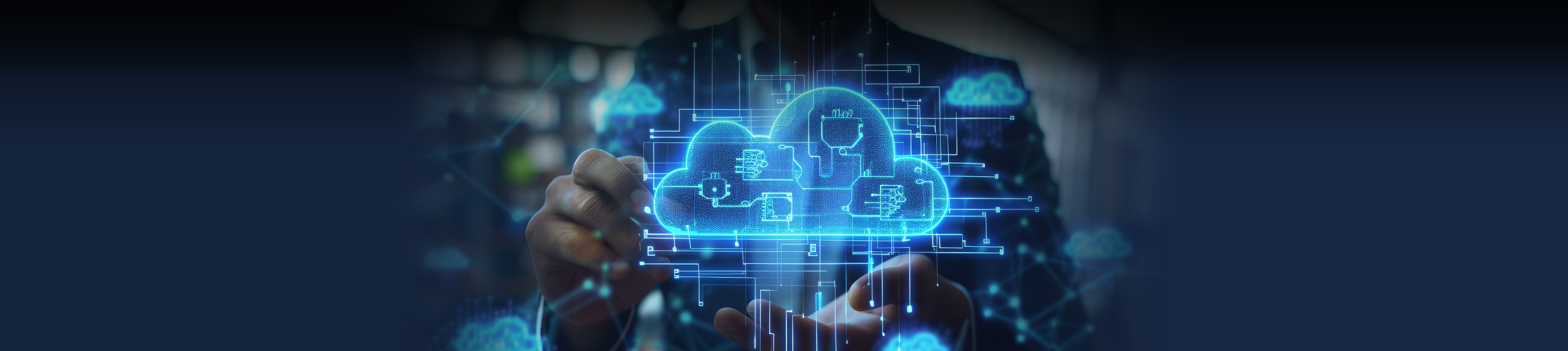
ACL Digital
10 Minutes read
Edge vs. Cloud: Choosing the Right ML Approach for Production Quality Control
In a world where quality can make or break a brand’s reputation, manufacturers are constantly looking for innovative ways to stay ahead of the competition, entering machine learning (ML), a game-changer in the realm of production quality control. However, the question remains: which ML approach will best serve your needs – edge computing or cloud computing?
Both edge and cloud-based ML solutions offer distinct advantages and come with their own set of challenges. Edge computing enables real-time data processing closer to the source, offering speed and reduced latency, which can be critical in time-sensitive production environments. On the other hand, cloud computing provides robust computational power and scalability, allowing manufacturers to process and analyze vast amounts of data.
What is DevOps?
Elevated Customer Satisfaction
Choosing the right deployment approach for machine learning in production quality control is a strategic decision with significant implications for efficiency, cost, and competitive advantage. Here’s why it matters:
Real-Time Responsiveness
Edge computing processes data locally, reducing latency and enabling real-time decision-making. This is crucial for applications like automated inspection systems where immediate action is needed. Cloud computing, while powerful, may introduce delays due to data transmission, making it less suitable for time-sensitive tasks but ideal for batch processing and analytics.
Scalability and Computational Power
Cloud computing offers immense scalability and computational power, allowing businesses to handle large datasets and complex models without infrastructure constraints. This is perfect for companies anticipating growth or dealing with massive data volumes. Edge solutions, while potentially limited in scalability, ensure consistent performance without relying on internet connectivity.
Data Privacy and Security
Edge computing enhances security by keeping sensitive data local, reducing exposure to cyber threats. This is crucial for industries with strict regulatory requirements. Conversely, cloud computing providers invest heavily in security measures, offering encryption and compliance certifications to protect data in transit and at rest.
Cost Considerations
Edge computing may require a higher initial investment in hardware, but it can lead to long-term savings by minimizing data transmission and recurring service fees. Cloud computing typically operates on a pay-as-you-go model, which is cost-effective for businesses looking to avoid significant upfront expenses.
Network Reliability and Connectivity
Edge computing is ideal for locations with unreliable internet connectivity, as it allows for continued operation independent of external networks. Cloud computing requires stable internet access but provides seamless integration with online services, facilitating data sharing and collaboration.
Adaptability and Future-Proofing
Edge solutions offer flexibility in integrating new devices and adapting to technological changes quickly. Meanwhile, cloud computing provides access to the latest technologies and updates, offering long-term benefits as new advancements emerge. Balancing immediate adaptability with future scalability is key.
Understanding Edge and Cloud Computing
The choice between edge and cloud deployment for machine learning in production quality control depends on specific needs and priorities. By considering factors such as responsiveness, scalability, security, cost, network reliability, and adaptability, manufacturers can make informed decisions that align with their goals and maximize the value of their ML initiatives. Choosing the right deployment approach can enhance quality standards, reduce costs, and maintain a competitive edge in the market.
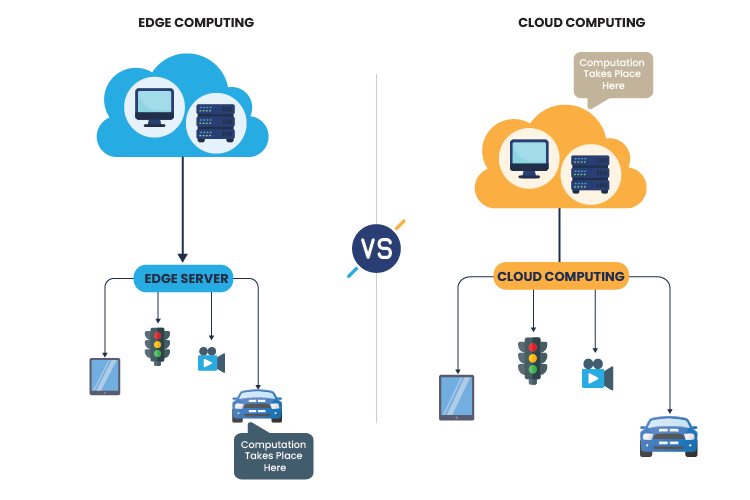
Edge and cloud computing have emerged as two pivotal approaches, each offering unique advantages and transforming the way businesses manage data and applications. As more organizations look to integrate machine learning (ML) and artificial intelligence (AI) into their operations, understanding these two computing paradigms is essential for making informed decisions. Let’s dive into what edge and cloud computing are and explore their key characteristics.
What is Edge Computing?
Edge computing is a decentralized computing architecture that brings computation and data storage closer to the data source or end-user. Instead of relying on a centralized data center, edge computing processes data on local devices or near the source of data collection, such as sensors, IoT devices, or local servers.
Key Characteristics of Edge Computing
Edge computing optimizes data processing by positioning it closer to the source, resulting in reduced latency and improved real-time responsiveness for applications like autonomous vehicles and industrial automation. This approach conserves bandwidth by minimizing data transfer to central servers, making it well-suited for IoT and video surveillance. Additionally, edge computing enhances system reliability by maintaining functionality during network disruptions and bolsters data security by keeping sensitive information local, which is critical for compliance in regulated industries such as healthcare and finance.
- Low latency is achieved by processing data locally, enabling real-time responses essential for autonomous vehicles.
- Edge computing conserves bandwidth by reducing data sent to central servers, which is beneficial for IoT and video surveillance.
- Reliability is improved as edge computing maintains operation during network outages, crucial for healthcare and emergency services.
- By keeping data local, edge computing enhances security and meets compliance requirements in industries like finance and healthcare.
What is Cloud Computing?
Cloud computing is a centralized model where data storage, processing, and management are conducted in remote data centers owned by cloud service providers. This model offers vast computational resources and is accessible over the internet, allowing businesses to leverage powerful tools and services without maintaining physical hardware.
Key Characteristics of Cloud Computing
- Scalability in cloud computing lets businesses easily adjust resources based on demand, making it perfect for handling fluctuating workloads.
- Cloud computing reduces capital expenses by eliminating the need for on-premises hardware, offering cost-effective pay-as-you-go pricing models.
- Global accessibility in cloud computing allows users to access data and applications from anywhere, supporting remote work and collaboration.
- Cloud providers offer robust security with features like encryption and authentication, ensuring data stored in the cloud is protected against threats.
- Cloud platforms offer advanced analytics and AI services that help businesses extract insights and drive innovation using powerful tools like machine learning.
ML in Manufacturing Production Quality Control
Machine Learning (ML) is revolutionizing the manufacturing industry, particularly in the realm of production quality control. By leveraging advanced algorithms and data analytics, ML enables manufacturers to enhance product quality, reduce defects, and streamline operations. Through predictive analytics, anomaly detection, and automated inspections, ML-driven systems can identify quality issues early in the production process, leading to significant cost savings and improved efficiency. With the ability to analyze vast amounts of data in real time, ML helps manufacturers make data-driven decisions, ensuring that products meet strict quality standards while minimizing waste and downtime. As a result, ML is becoming an essential tool for manufacturers aiming to maintain a competitive edge in a rapidly evolving market.
Benefits of Edge Computing for ML in Quality Control
Edge computing offers several advantages for implementing ML in quality control processes:
- Edge computing enables real-time analytics for instant quality issue detection, maintaining production efficiency and minimizing defects.
- Edge computing reduces latency by processing data locally, allowing for quick reactions in scenarios like fast-moving production lines.
- Only essential data is sent to the cloud, conserving bandwidth in high-volume environments like video surveillance and IoT devices.
- Local data processing enhances security and privacy, reducing exposure to cyber threats and meeting regulatory standards.
- Edge computing maintains ML model functionality during network disruptions, ensuring reliable quality control in areas with inconsistent connectivity.
Benefits of Cloud Computing for ML in Quality Control
Edge computing offers several advantages for implementing ML in quality control processes:
- Edge computing enables real-time analytics for instant quality issue detection, maintaining production efficiency and minimizing defects.
- Edge computing reduces latency by processing data locally, allowing for quick reactions in scenarios like fast-moving production lines.
- Only essential data is sent to the cloud, conserving bandwidth in high-volume environments like video surveillance and IoT devices.
- Local data processing enhances security and privacy, reducing exposure to cyber threats and meeting regulatory standards.
- Edge computing maintains ML model functionality during network disruptions, ensuring reliable quality control in areas with inconsistent connectivity.
Comparing Edge and Cloud ML Solutions
When evaluating ML solutions for quality control, manufacturers must weigh the benefits of edge and cloud computing:
Latency and Responsiveness
Edge computing excels in scenarios requiring low latency and rapid responses, making it ideal for real-time applications. In contrast, cloud computing may introduce delays due to data transmission but offers powerful analytical capabilities for non-time-sensitive tasks.
Scalability and Resource Availability
Cloud computing provides superior scalability, allowing manufacturers to handle extensive data processing and storage needs. Edge computing, while offering localized processing, may face scalability limitations due to hardware constraints.
Data Privacy and Security
Edge computing enhances privacy by keeping sensitive data local, reducing exposure to cyber threats. Cloud computing, however, offers robust security measures implemented by providers, ensuring data protection across distributed environments.
Cost Considerations
Edge computing may involve higher initial hardware investments, while cloud computing operates on a subscription or pay-as-you-go model, distributing costs over time.
Network Dependency
Edge computing ensures continued operation in areas with unreliable internet connectivity, while cloud computing relies on stable network connections to function effectively.
Choosing the Right Approach for Your Manufacturing Needs
Selecting the appropriate ML deployment approach depends on various factors:
- Consider if your applications need real-time processing, large-scale analytics, or enhanced security to choose the right solution.
- Assess your budget and current infrastructure to weigh initial edge computing investments or ongoing cloud service costs.
- Evaluate data sensitivity and compliance needs to select solutions with the required security and privacy measures.
- Analyze network connectivity at your sites to decide if a hybrid approach is needed for varying conditions.
Consider long-term goals and scalability to ensure the chosen approach can adapt to future growth and technological advancements. Machine learning is transforming quality control in manufacturing by enabling real-time insights and predictive analytics.
ACL Digital’s ML offerings provide cutting-edge solutions tailored to both edge and cloud environments, leveraging advanced algorithms to enhance operational efficiency. Both edge and cloud computing offer unique advantages for deploying ML solutions, and the choice between them should be guided by specific application requirements, budget considerations, and data security needs.
A hybrid approach that combines the strengths of edge and cloud computing can offer manufacturers a flexible and scalable solution, optimizing operations and maintaining a competitive edge. By carefully evaluating their needs and priorities, manufacturers can leverage ACL Digital’s ML capabilities to drive quality improvements, enhance efficiency, and achieve business success. For more details reach out to the experts at business@acldigital.com.
Related Insights
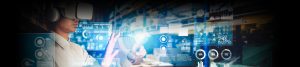
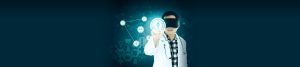
AI-Powered Personalization in UX: Enhancing User Experience in Healthcare
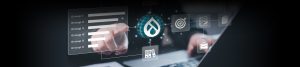
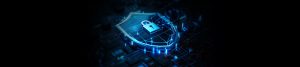
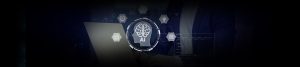
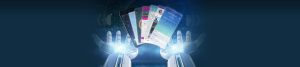